How do we rate carbon credits? The Sylvera Carbon Credit Rating process
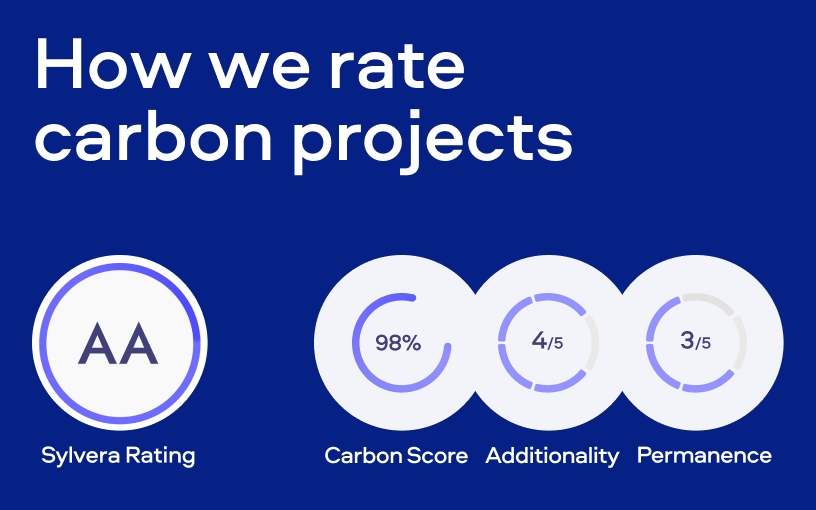
The Sylvera carbon credit rating creation process consists of two stages:
- Stage 1: the development of a robust project-type-specific rating framework
- Stage 2: the application of this framework to an individual project to create a Sylvera carbon credit rating
You can download our white paper, which describes these stages and our overall methodology here.
Stage 1: Developing a project-type-specific framework
What are Sylvera carbon credit rating frameworks?
Sylvera ratings are created by first developing a proprietary framework for assessing a specific type of carbon project, such as reducing deforestation and forest degradation (REDD+), afforestation, reforestation and revegetation (ARR), improved forest management (IFM) or deploying renewables in place of carbon-intensive forms of electricity generation.
We develop frameworks based on individual project types, rather than a highly generalized framework, because different projects have a diversity of activities and incentives that need to be assessed in a distinct way to gain in-depth insights into the project’s quality. A general framework, we believe, would not reflect the nuances of individual project performance, resulting in inaccurate ratings and ultimately reducing confidence in carbon projects and carbon markets.
Our frameworks are rooted in the relevant carbon crediting methodologies and characteristics of the project type at hand. They are sensitive to revealing the key features and issues of a certain project type. We design them to be fair and impartial in their treatment or judgment of carbon projects, and provide consistent and comparable quality metrics that can be used to make up our scoring pillars which apply to carbon projects across frameworks.
Diversity across project types
Activities
Different projects implement different activities. For example, some nature-based projects protect existing forests while others seek to reforest areas by planting trees. This has huge implications for the way the GHG avoidance or removal of the project is quantified. The former requires monitoring of forest loss, while the latter requires monitoring of planting areas and growth rates of new trees.
Different project types have varying incentives. This has meaningful implications for the additionality of projects, whether the projects are of the same type or of different project types. For example, a project that protects existing forests relies more heavily on the finances provided by the sale of carbon credits because it doesn’t have the same access to the revenue a large renewable project can generate from selling electricity.
Incentives
The existence of carbon projects can also create perverse incentives. For reforestation projects, this can manifest in the conversion of native ecosystems for the purpose of developing a carbon project. For these project types, Sylvera conducts an independent assessment of the land use and land cover change of the project area prior to project start.
Developing the framework
The development of a project-type-specific framework takes between 1,500 to 2,500 hours to complete. As we progress, this process is becoming increasingly streamlined, allowing us to move at greater speed. It involves six steps.
1. Discover
We conduct initial research into a project type and identify key quality indicators under our scoring pillars that are specific to this project type. Our team assesses relevant carbon project type certification methodology documentation from carbon credit registries, such as Verra or Gold Standard, reviews documentation from sample projects and reads academic papers and industry publications. We also explore technical requirements, capabilities and challenges specific to the project type that must be addressed to arrive at a robust quality assessment.
2. Define
We then define the what, why and how of the framework subcomponents and questions. For each component, we identify required data sources and define the analysis necessary to provide a holistic and rigorous assessment. Our framework principles, rationale and scoring logic are then presented to our internal stakeholder committee representing diverse subject matter experts, many of whom interact with both policy and commercial partners, for feedback. The new framework is then applied to a sample set of 30 projects and assessed by our ML, geospatial, data extraction and ratings analyst teams.
3. Scope
In the scope phase, we assess the work required to productionize the process of rating carbon credits using this framework. This includes defining the requirements and deliverables for developing automated workflows for data outputs from the ML and geographic information science (GIS) teams, as well as mapping production processes and defining documentation requirements. We also work with our quality assurance (QA) team to embed processes that ensure consistency and accuracy in Sylvera ratings.
4. Iterate
Feedback from the internal stakeholder committee is integrated into the framework and the required models are built so that testing of a sample of initial ratings can commence. These samples are used to test the logic of the new framework. This includes fine-tuning the weights of our scores and our scoring matrices, which are sets of rules for how our scores interact with one another to arrive at a Sylvera rating. If they arise, we also integrate any possible outputs that occur at extreme ends of the spectrum, known as corner cases. We also run our feedback by our customer council. This consultation process allows us to gain early insights into the value of our new framework.
5. Train
The framework development team implements a framework training curriculum to educate and train the production team on the mechanics of implementing the project type framework. The production team then begins to populate Sylvera scores closely guided by the framework team. Unexpected results, special cases, process improvements and any scores that diverge too much from the norm are discussed.
6. Deploy
Our framework is signed off and ready to be used to create publishable Sylvera carbon credit ratings. The framework and corresponding documentation are completed and communicated with our production team.
Our framework development roadmap
Because we believe that we need to develop project-type-specific frameworks first, we had to choose which type of project with which to start. We chose avoided unplanned deforestation (AUD) REDD+, because nature-based credits account for the lion's share of VCMs, with AUD REDD+ accounting for a significant portion. Many of these nature-based carbon credits are currently being purchased to meet climate commitments with limited visibility and understanding of their quality and performance.
Unclear performance of projects and opaque baseline modeling created an opportunity to provide rigorous assessments of quality that help direct capital toward high-quality projects while also limiting the use of ineffective credits to support claims of climate action.
Our team is currently in the process of developing new frameworks to assess the quality of the majority of available credits, regardless of project type.

Stage 2: The credit rating process
Once a rating framework has been developed, our team can get started producing ratings for individual projects. Initially, the project rating process takes between 60 to 120 hours depending on the complexity and nuances of the project. However, this too is becoming more streamlined as we build more automation into the process. Our team conducts an in-depth bottom-up analysis of project specifics including primary data on performance, and a top-down assessment of risks that the project is exposed to.
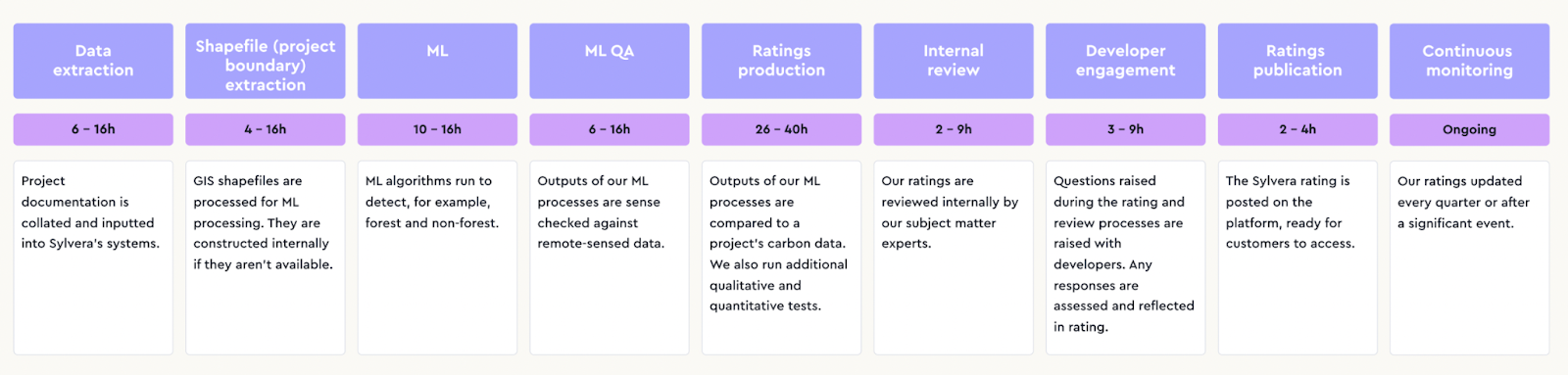
1. Data extraction
All relevant data points required to assess the quality of a project are extracted from the publicly available project documentation published by carbon credit registries and other public sources of information, including academic literature and evidence-backed press coverage. A significant portion of this process is automated, but support from our in-house data extraction team is required due to inconsistencies in document structure and reporting. Our team reads through hundreds of pages of project documentation so you don’t have to.
2. Shapefile (project boundary) extraction
If relevant, shapefiles of the project boundaries are extracted or are constructed by our team if not provided. This enables us to ensure that, for example, any monitoring of forest gain or loss is conducted within the exact boundaries of the project with a high degree of accuracy. Our GIS specialists also investigate local project characteristics that may require additional care during the ML process — such as areas with heavy cloud cover or highly seasonal biomes — to reduce the likelihood of misclassifying forest gain or loss.
3. ML
We have developed proprietary ML models in-house to monitor specific aspects of carbon projects, for example, forest cover over time in a range of biomes. These are used to track and compare actual emissions with those reported by the project and feed directly into our carbon score. We also track trends, such as deforestation over time prior to the project start date and ongoing since, to enable us to verify whether the claimed threats to the project are real and whether the magnitude of risk stated has materialized in nearby, similar areas.
4. ML QA
QA is important to make sure that the outcomes of our ML models are accurate. We internally verify the ML model classifications of forest parameters — such as canopy cover — using peer-reviewed standard metrics and comparison with additional data sources. These processes, along with accuracy assessments conducted on over 500 points per project area by leveraging our GIS team’s expertise and optical satellite data, are used to identify the potential errors of the classification and quantify the uncertainty of these estimates.
5. Ratings production
The individual pillar scores of our rating are compiled by applying our rating frameworks to available information on the project to develop a preliminary rating. The available information includes the extensive project data extracted and cleaned from the public registry documentation, other project and country contextual data collated from verified external sources, proprietary ML outputs using satellite imagery and multiple GIS open-source datasets.
6. Internal review
A thorough review and rigorous discussion of the preliminary rating are conducted by our subject matter experts. While our ratings process has been designed to be as objective as possible, this qualitative review is key to ensuring the rating appropriately reflects the quality of the project.
7. Developer engagement
Unlike many rating providers, we maintain our independence by not accepting payments from developers to rate their projects. However, we believe it is critical to engage with developers throughout the rating process to secure additional information required to accurately rate a project and give developers the right of reply and the opportunity to provide additional evidence.
8. Ratings publication
Once the rating has passed internal review and reflects any additional information provided by the developer, we publish our assessment on our platform. This includes a rating, individual subscores, the underlying commentary and rationale that supports our analysis, our maps of projects, pricing data from Xpansiv CBL and issuance data from carbon credit registries.
9. Continuous monitoring
Every quarter, for every project, we input the most recent satellite data into our ML models to capture potential changes in carbon stock (for example, deforestation or growth). We also re-scrape data from registries to gather recent reports and issuance data, as well as any other public information that might be relevant. Significant events such as fires, changes in the project proponent team structure, or the release of significant information, will trigger an ad-hoc reassessment of the project.
What data do we input, analyze and output?
Input
- Carbon credit registries like Verra, Gold Standard and others
- Optical, light detection and ranging (lidar) and synthetic aperture radar (SAR) satellite
- Forest databases like Global Forest Watch, Hansen et al Global Forest Change Data and others
- Infrastructure, settlement and land use data from OpenStreetMap, Spatial Database of Planted Trees and the United States Geological Survey (USGS)
- Protection and biodiversity status provided by the Integrated Biodiversity Assessment Tool (IBAT)
- Active fire monitoring from the National Aeronautics and Space Administration’s (NASA’s) Fire Information for Resource Management System (FIRMS)
- World Bank and Food and Agriculture Organization (FAO)
- National and regional policy and regulation documentation
- Carbon credit exchange platform, CBL Xpansiv
- Emission Reductions Payment Agreements (ERPA) and long-term offtake agreements
- Academics papers and industry research
Analysis
- Proprietary ML models
- GIS analysis
- Our proprietary ratings frameworks
Output
- Sylvera rating, carbon score, additionality score, permanence score
- Co-benefits score
- Detailed discussions of our rationale for each element of the score
- Summary of project context
- Maps, if relevant
- Carbon credit price and carbon credit issuance analytics
Learn more about why we created this proprietary carbon credit rating system here and download the complete white paper detailing our processes here.
Learn how businesses are shaping carbon market strategies with carbon.

The end-to end carbon dataplatform for the 'net' in yournet zero goals
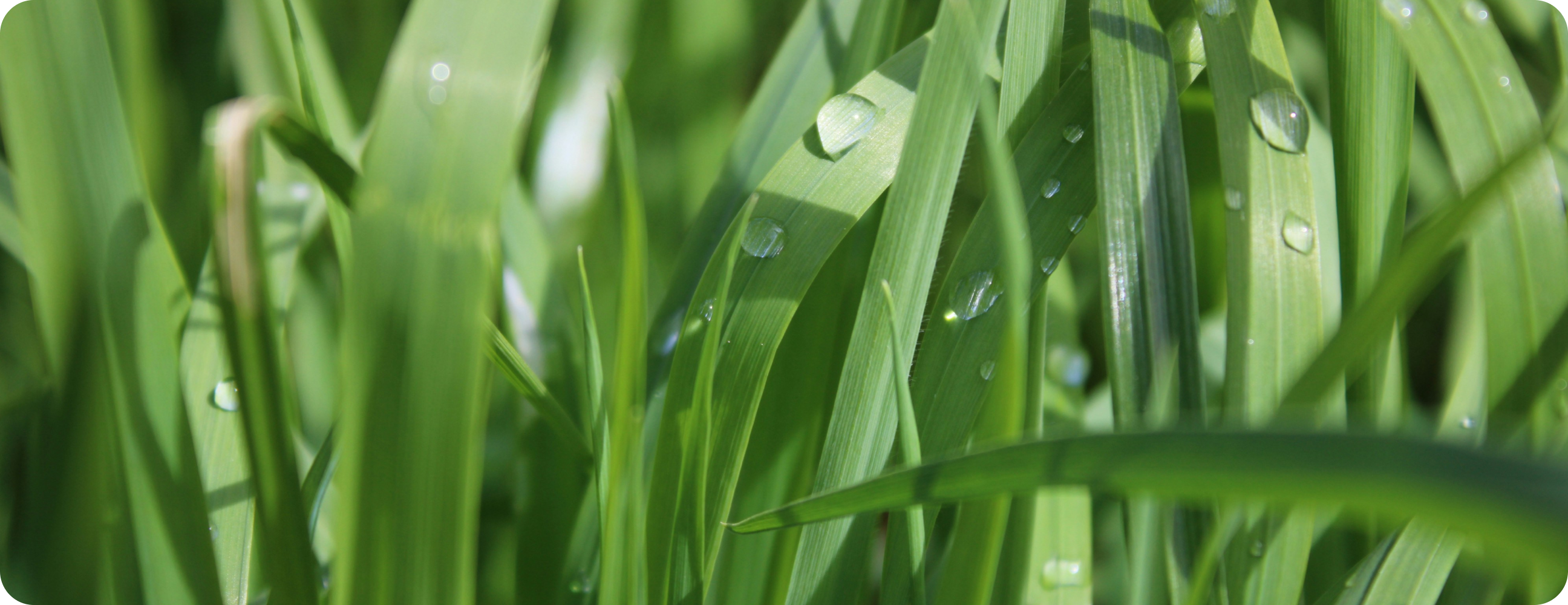